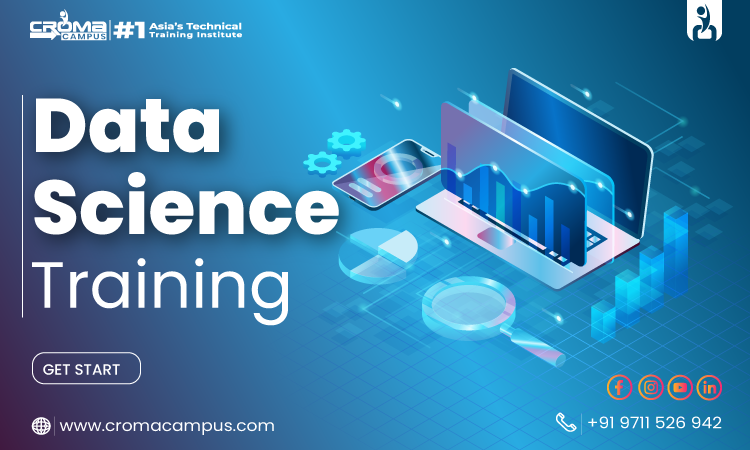
What Is The Difference Between Data Science And Full Stack Data Science?
Data Science is a multidisciplinary field that focuses on extracting insights and knowledge from data. It involves various stages, including data collection, data cleaning, exploratory data analysis (EDA), feature engineering, modelling, and data visualization. In contrast, Full Stack Data Science expands on the traditional Data Science role. It encompasses the entire data pipeline, from data acquisition to deploying data-driven solutions in a production environment. The Data Scientists leverage statistical techniques, Machine Learning algorithms, and domain knowledge to make data-driven decisions and solve complex problems. However, Full Stack Data Scientists have the analytical skills of a Data Scientist alongside engineering expertise. The Full Stack Data Science Course helps you learn more about the roles of Full Stack Data Scientists. Data Science is an evolving technology, and training in this technology can open doors to numerous career opportunities.
How Are Data Science And Full Stack Data Science Different?
Data Science and Full Stack Data Science are related fields but have some key differences. Here is a detailed differentiation between Data Science and Full Stack Data Science.
Scope And Depth
- Data Science: Data scientists usually specialize in one or more aspects of the data analysis process. Some may focus on predictive modelling, while others excel in data visualization or data cleaning. Their expertise tends to be more concentrated within the realm of data analysis.
- Full Stack Data Science: Full Stack Data Scientists have a wider scope of expertise. They are proficient not only in data analysis but also in data engineering and deployment. They can handle the entire data lifecycle, from collecting raw data to deploying machine learning models in production environments.
Skills Required
- Data Science: Data scientists require strong analytical skills, statistical knowledge, and proficiency in programming languages like Python or R. They often work with libraries and tools such as pandas, scikit-learn, and Jupyter notebooks to conduct their analyses.
- Full Stack Data Science: Full Stack Data Scientists need a more diverse skill set. In addition to data analysis and machine learning, they should be skilled in data engineering, database management, web development, and potentially cloud computing. They may work with tools like Apache Spark, SQL databases, Docker, and web frameworks like Flask or Django.
Responsibilities
- Data Science: Data scientists primarily focus on data analysis, modelling, and generating insights. Their primary goal is to extract meaningful information from data and use it to inform business decisions or solve specific problems.
- Full Stack Data Science: Full Stack Data Scientists have a broader range of responsibilities. They are responsible for acquiring and preprocessing data, designing and implementing data pipelines, creating machine learning models, deploying models as APIs or services, and monitoring and maintaining these services in production environments.
Project Lifecycle
- Data Science: Data science projects often involve exploratory data analysis, feature engineering, model training, and evaluation. The results are typically communicated through reports, visualizations, or presentations.
- Full Stack Data Science: Full Stack Data Science projects cover the entire data lifecycle. They start with data collection and ingestion, move through data cleaning and transformation, model development, deployment of models in real-world applications, and ongoing maintenance to ensure the models perform effectively over time.
Employment Roles
- Data Science: Data scientists typically hold job titles like Data Analyst, Machine Learning Engineer, or Data Scientist. Their roles revolve around data analysis and modelling.
- Full Stack Data Science: Full Stack Data Scientists may have titles such as Data Engineer, Full Stack Data Scientist, or Machine Learning Engineer with Full Stack skills. They are responsible for end-to-end data solutions, from data infrastructure to deploying and maintaining data-driven applications.
Conclusion
In conclusion, Data Science and Full Stack Data Science share a foundation in data analysis. Full Stack Data Scientists take on a broader set of responsibilities that encompass the entire data pipeline and require expertise in both data analysis and data engineering. Numerous Noida-based companies greatly hire skilled Data science and Full Stack Data Science professionals. Therefore, joining the Data Science Training Institute in Noida helps you develop all the industry-relevant skills, and get hired quickly. The roles of Data scientists are crucial in today’s data-driven world. These professionals bridge the gap between data analysis and practical application, enabling organizations to derive maximum value from their data.