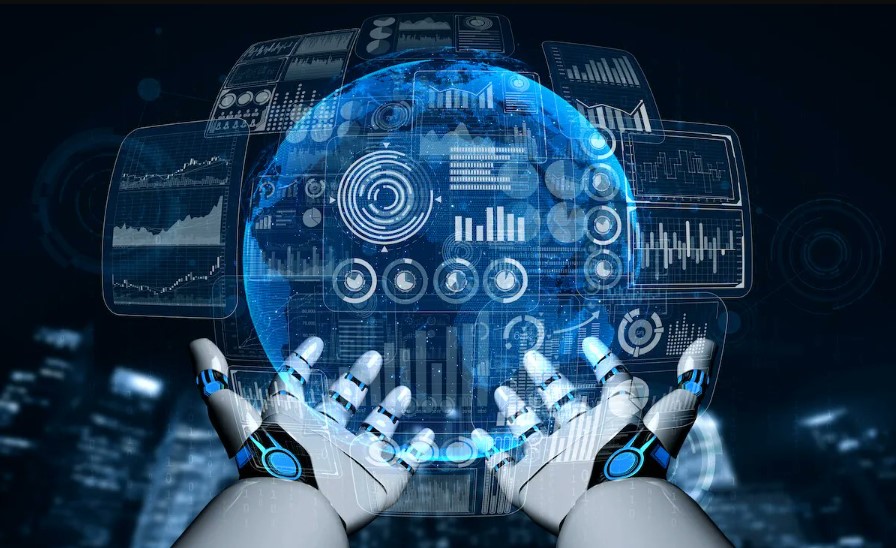
SYNTHETIC DATA: THE COMPLETE GUIDE
A comprehensive guide to synthetic data covers various aspects of synthetic data generation, its applications, challenges, and best practices. Here’s an overview of what a complete guide to synthetic data might include:
1. Introduction to Synthetic Data:
- Definition and concept of synthetic data.
- Reasons and benefits of using synthetic data.
2. Applications:
- Discuss real-world applications in various fields, such as healthcare, finance, machine learning, and more.
3. Generating Synthetic Data:
- Techniques and methods for generating synthetic data, including:
- Randomization
- Generative Models (e.g., GANs and VAEs)
- Rule-Based Generation
- Data Masking and Perturbation
- Interpolation and Extrapolation
4. Data Privacy and Security:
- The role of synthetic data in preserving privacy and security.
- Comparison with data anonymization and de-identification.
5. Data Quality and Limitations:
- Discuss the quality of synthetic data compared to real data.
- Limitations and challenges in synthetic data generation.
6. Use Cases:
- Explore specific use cases where synthetic data is valuable, such as:
- Privacy-preserving analytics
- Machine learning model development
- Simulation and testing
- Data augmentation
7. Legal and Ethical Considerations:
- Address the legal and ethical aspects, including compliance with data protection regulations (e.g., GDPR) and ethical guidelines.
8. Best Practices:
- Tips and best practices for effectively using synthetic data, including quality assessment, data validation, and model evaluation.
9. Tools and Frameworks:
- An overview of software tools and frameworks that facilitate synthetic data generation.
10. Challenges and Future Trends: – Discuss ongoing challenges and emerging trends in the field of synthetic data, such as improving data quality and addressing ethical concerns.
11. Case Studies:
- Real-world examples and case studies showcasing how organizations have successfully employed synthetic data.
12. Resources:
- Provide references to books, research papers, websites, and tools for further learning and implementation.
13. Glossary:
- Define key terms and concepts related to synthetic data.
14. Conclusion:
- Summarize the importance of synthetic data in the modern data landscape and its potential for addressing privacy concerns and data scarcity issues.
Such a comprehensive guide to synthetic data would be valuable for data scientists, researchers, privacy professionals, and anyone working with data that requires privacy protection or data augmentation. It would help them understand the principles, techniques, and best practices associated with synthetic data generation.